AI’s power and promise: balancing innovation, sustainability and data integrity for climate action
By: Emilie Sartoretto and Tom Lebrun
Artificial Intelligence – AI – is transforming industries by offering innovative solutions for climate monitoring, biodiversity conservation and energy efficiency. By analyzing massive datasets, AI has the potential to drive sustainability efforts on a global scale.
However, this progress comes at a cost. The growing energy and water consumption required to power data centers, coupled with the vast computing demands of AI models like GPT-3, has a significant environmental impact. Training such models can require the same amount of electricity needed to power 130 U.S. homes for a year, adding pressure to already strained energy grids and contributing to greenhouse gas emissions.
Also, AI’s reliance on high-quality data is fundamental to its effectiveness. Poor data leads to flawed models, undermining AI’s potential to address critical issues like climate change. As AI adoption expands, so does its environmental footprint and ensuring the data that powers AI is accurate, consistent and reliable is key to maximizing its positive impact.
This is where the role of standards and resource-efficient AI comes into play, helping to balance AI’s promise with its resource consumption, while ensuring AI models are built on a foundation of trustworthy, standardized data.
Frugal AI: addressing resource challenges
With AI’s increasing environmental demands, a new approach—frugal AI—is emerging to design AI systems that are both efficient and environmentally responsible. Frugal AI focuses on minimizing resource consumption while maximizing the benefits AI can deliver.
Standards like AFNOR Spec 2314 on Frugal AI provide best practices for reducing AI’s environmental footprint, emphasizing energy efficiency, moderation by design and optimizing AI models for sustainable performance.
This approach pushes AI developers to carefully assess whether AI is the best tool for a given problem, to find ways to use AI more efficiently and to reduce the overall carbon and water footprint of AI systems. By integrating frugal AI practices, we can ensure that AI innovation is aligned with sustainability goals and minimize the trade-offs between progress and environmental impact.
Standards and data: the foundation of effective AI solutions
The success of AI in climate action depends not only on its technology but on the quality of the data it uses. Poor data can lead to flawed AI models, resulting in ineffective or even harmful decisions. Standards ensure that the data used in AI systems is reliable, consistent and fit for purpose—building trust in AI-driven climate solutions.
One strong example of how standards ensure high-quality data is the collaboration between SCC and Environment and Climate Change Canada (ECCC) to improve Canada’s weather monitoring systems. Accurate weather data is crucial not only for designing infrastructure that can adapt to a changing climate but also for enabling AI models to deliver more precise predictions.
As demand for localized meteorological data has grown—with provinces, municipalities and academic organizations contributing—SCC supported the development of a four-part series of standards to ensure the consistency and reliability of weather data collected across Canada.
These standards address key elements such as metadata, site selection, instrument installation and data quality ratings, ensuring that data from thousands of weather stations meet standardized criteria. This collaboration also aligns with ECCC’s Artificial Intelligence Integration Roadmap, which integrates AI into their numerical weather and environmental prediction systems, highlighting the importance of high-quality data for improving AI model accuracy and climate resilience efforts.
Other key international and national standards play a similarly vital role in ensuring data quality for AI systems used in climate action, including:
- ISO 14064-1:2018 Greenhouse Gases – Requirements for quantifying and reporting greenhouse gas emissions and removals, crucial for managing climate data.
- ISO 14090:2019 Adaptation to climate change – Principles and guidelines for managing climate adaptation, emphasizing reliable data for risk assessments.
- World Meteorological Organization (WMO) Data Sharing Guidelines: Global standards for sharing meteorological, hydrological and climate data in real-time, ensuring data reliability for climate models and early warning systems.
- CSA Group’s hydrometeorological monitoring station data standards: Ensures consistent data collection and sharing from weather stations across Canada, supporting more accurate climate monitoring and response.
These standards provide the foundation for reliable, consistent data that AI systems depend on for climate action. By standardizing data collection and management, they enable AI-driven solutions to work effectively and support global sustainability goals.
Case studies: AI-driven innovation for climate action
Several AI-driven projects in Canada demonstrate the potential of AI to tackle climate change and sustainability challenges, illustrating the critical role of high-quality data and standards. These projects underscore how AI, combined with robust data frameworks, can drive impactful climate solutions.
Researchers at Université de Moncton developed an AI tool that generates life-like birdsongs to help ecologists monitor rare bird species. By expanding datasets of underrepresented species, this tool enhances AI models for acoustic monitoring. Standardized data collection practices improve the reliability of these tools, making them more effective for biodiversity conservation.
Similarly, the Antenna Insect Data Platform, developed by MILA, uses machine learning to monitor insect populations globally. By employing high-resolution cameras and AI-based methods, researchers are better equipped to address climate and biodiversity crises. Applying data standards ensures AI tools like these work effectively across diverse ecosystems and research teams.
Another standout project from the University of Toronto accelerates the discovery of clean energy materials using AI. AI models rapidly analyze large datasets to identify new materials for batteries and solar cells. The success of this initiative depends on data integrity and applying global standards helps achieve scalable outcomes for clean energy solutions.
These projects highlight AI’s promise in climate action but also emphasize the importance of high-quality data and standards in maximizing impact. Integrating standards into AI projects supports innovation while aligning with climate and sustainability goals.
What now?
While standards are essential, they are only part of the solution. Ensuring the consistent and correct application of standards is just as important. Accredited conformity assessment plays a critical role in making sure standards are applied properly, ensuring transparency, trust and credibility in climate action efforts.
One notable example is SCC’s Verification and Validation Accreditation Program, which ensures the accuracy and reliability of climate action claims by validating future sustainability efforts and verifying past environmental statements. This process is key to distinguishing genuine efforts from greenwashing and ensuring clear reporting.
The Clean Fuels Regulations (CFR) verification scheme is a prime example of this program in action. Established in partnership with ECCC, the CFR promotes clean technology and low-carbon fuel use across Canada by setting greenhouse gas reduction targets and introducing a credit market for compliance. SCC accredits the verification bodies responsible for assessing the required reports under the regulation, helping drive innovation, reduce emissions and promote clean technology adoption across the country.
Accredited verification and validation ensure that the data underlying sustainability efforts is both accurate and trustworthy. This connection between verified data and AI reinforces how standards, accreditation and quality assurance are essential for achieving climate goals and maximizing AI’s potential as a force for good.
Get involved
Are you an expert in software development or digital services? Your insights are needed.
DIN has launched an ISO Open Consultation to explore how software can become more resource-efficient, reducing its environmental impact. This is your chance to shape the global approach to resource-efficient software and contribute to recommendations for ISO.
Don’t miss the opportunity to make an impact—register now and join the discussion. Knowledge of standardization is helpful but not required.
Register before the deadline on November 11, 2024
About the authors
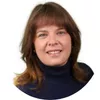
Emilie Sartoretto is a marketing and communications advisor at the Standards Council of Canada, working on the initiatives mentioned in this article including topics like the digital economy.

Tom Lebrun is a senior policy analyst at the Standards Council of Canada specializing in AI governance, regulation and international standardization, and an expert member for Canada on the ISO/IEC JTC1 SC 42 – Artificial Intelligence.